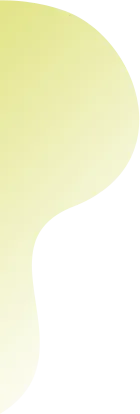
ArangoML Part 1: Where Graphs and Machine Learning Meet
Estimated reading time: 4 minutes
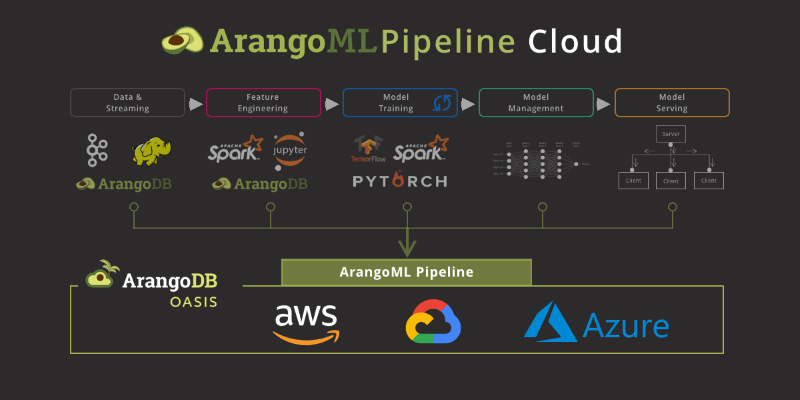
This post is the first in a series of posts introducing ArangoML and showcasing its benefits to your machine learning pipelines. In this first post, we look at what exactly ArangoML is, with later posts in this series showcasing the different tools and use cases.
If you have a use case you would like to see highlighted as a part of this series, please let us know on the ArangoML Slack channel.
Posts in this series:
ArangoML Part 1: Where Graphs and Machine Learning Meet
ArangoML Part 2: Basic Arangopipe Workflow
ArangoML Part 3: Bootstrapping and Bias Variance
ArangoML Part 4: Detecting Covariate Shift in Datasets
ArangoML Series: Intro to NetworkX Adapter
ArangoML Series: Multi-Model Collaboration
Overview
ArangoML is a set of tools and technologies that enable analytics and machine learning on graph data.
ArangoML Tools/Stack
The ArangoDB tool stack for analytics allows you to explore relationships of interest in your ArangoDB graph. These include:
- AQL: The ArangoDB Query Language is a declarative language that aims to be human-readable and supports the complex query patterns that are only possible within a multi-model database. These features make machine learning tasks more powerful and accessible to both the data scientists performing exploratory data analysis and the dev-ops personnel running ad hoc queries for maintenance and issue investigation. See the AQL Fundamentals for an overview of AQL and some examples of using AQL to query graphs in ArangoDB.
- Pregel: Data engineers use Pregel, a system for large-scale graph processing, to implement computationally intensive data transformations, while data scientists use it for data analysis tasks on large graphs. See our pregel tutorial for examples and an overview of using Pregel with ArangoDB.
- ArangoDB-NetworkX Adapter: NetworkX is a widely used tool for graph analytics; it provides robust implementations of many algorithms used for graph analytics. If your application requires such algorithms, you can leverage the implementation of these algorithms’ for your ArangoDB graphs. We provide an adapter to convert ArangoDB graphs to NetworkX graphs. You can leverage ArangoDB’s robust, reliable, and high-performing storage solution with the wide range of algorithms for graph analytics in NetworkX. We will cover our NetworkX implementation in greater detail in our upcoming network analytics post, be sure to sign up for our newsletter to be notified on the upcoming posts in this series. If you would like to explore some of the existing notebooks, you can find more examples in the repository.
Learning Through Metadata
In contrast to exploring relationships or entities in a graph, machine learning applications learn autonomously and continuously with new data. A key idea in making this possible is the use of machine learning pipelines. They make it possible for applications to learn from new data. Managing machine learning pipelines is achieved through metadata.
Metadata describes the components and actions involved in building the machine learning pipeline. The steps involved in constructing the pipeline are expressed as a graph by most tools, making ArangoDB a natural fit to store and manage machine learning application metadata. Arangopipe is ArangoDB’s tool for managing machine learning pipelines. Machine learning libraries such as DGL accept NetworkX graphs as input. Therefore the ArangoDB-Networkx adapter can be used to develop machine learning applications with ArangoDB graphs using libraries such as DGL. An example of using this available here.
Knowledge Graphs
Thanks to the features that come with using a multi-model database, it is possible to work with Knowledge Graphs(KGs) in ArangoDB; this combines the benefits of still being machine-readable while having the human readability benefits of a property graph. If you would like to learn more about using KGs with ArangoDB take a look at the upcoming Knowledge Graphs in ArangoDB series.
Stay Tuned
Be sure to sign up for our newsletter to be notified of the follow-up posts in this series!
This article is the first in a series covering the many different features of ArangoML. In the upcoming series, we will showcase topics such as:
- Using ArangoML for common network analysis tasks such as discovering structures.
- Using ArangoML to develop graph neural networks on graphs stored in ArangoDB.
- Using ArangoML for common tasks associated with model management.
Hear More from the Author
Introduction to ArangoDB ArangoGraph
Access Control with ArangoDB ArangoGraph
Continue Reading
Say Hi To ArangoDB ArangoGraph: A Fully-Managed Multi-Model Database Service
How we built our managed service on Kubernetes
ArangoDB Hot Backup – Creating consistent cluster-wide snapshots
Get the latest tutorials, blog posts and news: